Catalogue Search | MBRL
نتائج البحث
MBRLSearchResults
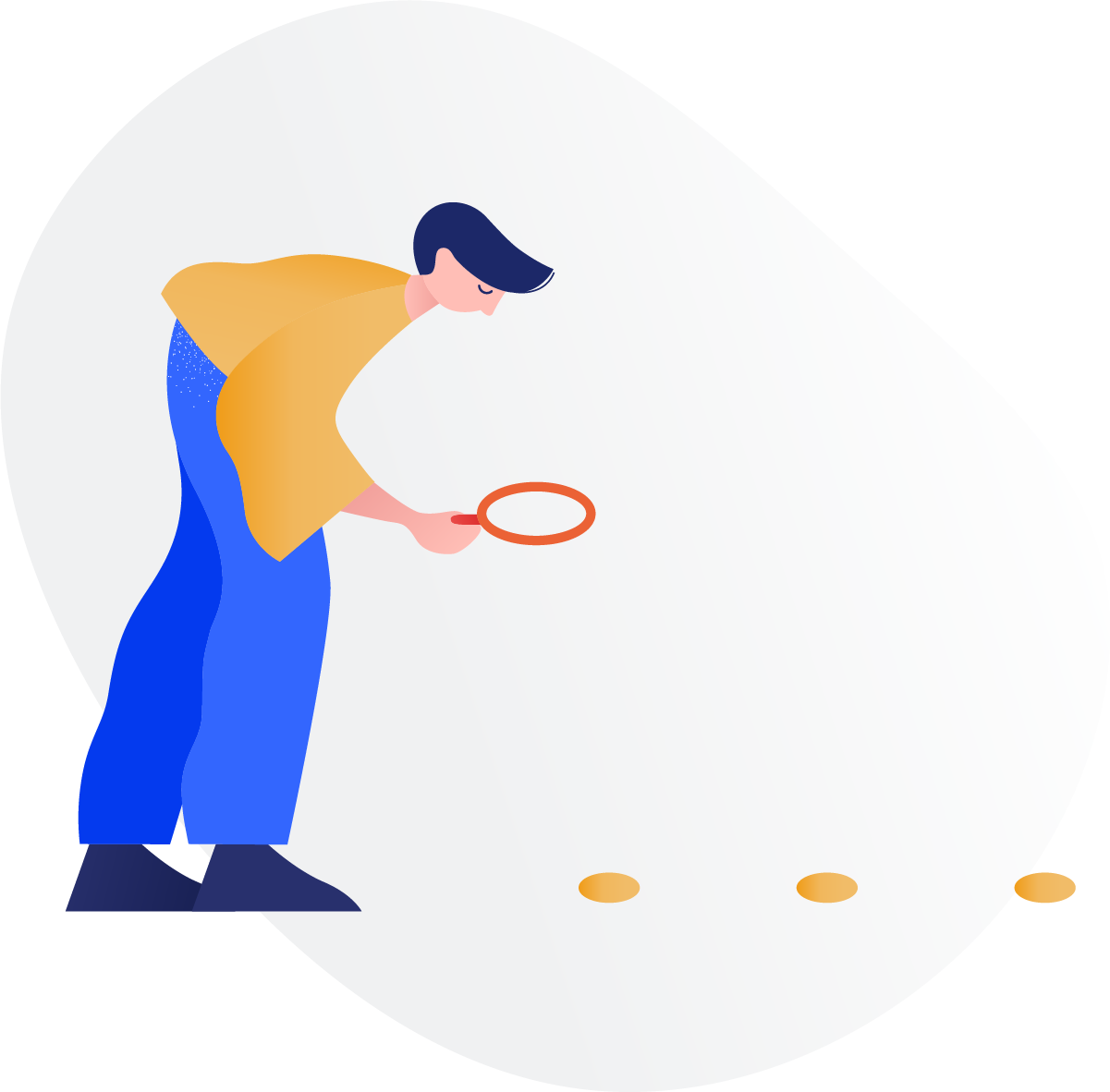
وجه الفتاة! هناك خطأ ما.
أثناء محاولة إضافة العنوان إلى الرف ، حدث خطأ ما :( يرجى إعادة المحاولة لاحقًا!
-
الضبطالضبط
-
مُحَكَّمةمُحَكَّمة
-
نوع العنصرنوع العنصر
-
الموضوعالموضوع
-
السنةمن:-إلى:
-
المزيد من المرشحاتالمزيد من المرشحاتالمصدراللغة
منجز
مرشحات
إعادة تعيين
5
نتائج ل
"Long, Zhiru"
صنف حسب:
Effects of Policy for Controlling Agricultural Non-Point Source Pollution in China: From a Perspective of Regional and Policy Measures Differences
بواسطة
Hua, Chunlin
,
Zhang, Jiuhong
,
Long, Zhiru
في
Agricultural industry
,
Agriculture
,
Analysis
2023
The concerns about the contradiction between agricultural production and Agricultural Non-Point (ANPS) pollution has become increased with economic development in China. Government interventions are key to controlling ANPS pollution through the establishment of laws and policies. This paper uses the entropy method to calculate the emissions amount of ANPS pollution and policy strength of 31 provinces from 2010 to 2019 in China. The dynamic panel data model with system generalized moment is used to estimate the impacts of policies with different measures on ANPS pollution emission. According to our findings, China's policies have been helpful in controlling ANPS pollution though there are important regional differences. Moreover, four types of policy measures all contribute to the reduction in ANPS pollution. These findings improve our understanding of the relationship between policies and ANPS pollution in the analyzed period, thus providing support for the formulation of pollution management strategies in the next stage.
Journal Article
W-GPCR Routing Method for Vehicular Ad Hoc Networks
2020
The high-speed dynamics of nodes and rapid change of network topology in vehicular ad hoc networks (VANETs) pose significant challenges for the design of routing protocols. Because of the unpredictability of VANETs, selecting the appropriate next-hop relay node, which is related to the performance of the routing protocol, is a difficult task. As an effective solution for VANETs, geographic routing has received extensive attention in recent years. The Greedy Perimeter Coordinator Routing (GPCR) protocol is a widely adopted position-based routing protocol. In this paper, to improve the performance in sparse networks, the local optimum, and the routing loop in the GPCR protocol, the Weighted-GPCR (W-GPCR) protocol is proposed. Firstly, the relationship between vehicle node routing and other parameters, such as the Euclidean distance between node pairs, driving direction, and density, is analyzed. Secondly, the composite parameter weighted model is established and the calculation method is designed for the existing routing problems; the weighted parameter ratio is selected adaptively in different scenarios, so as to obtain the optimal next-hop relay node. In order to verify the performance of the W-GPCR method, the proposed method is compared with existing methods, such as the traditional Geographic Perimeter Stateless Routing (GPSR) protocol and GPCR. Results show that this method is superior in terms of the package delivery ratio, end-to-end delay, and average hop count.
Journal Article
Retraction: Li, M., et al. W-GPCR Routing Method for Vehicular Ad Hoc Networks. Sensors 2020, 20 , 3406
2021
The journal retracts the article [...].
Journal Article
SimDCL: dropout-based simple graph contrastive learning for recommendation
بواسطة
Xu, YuHao
,
Guo, YunLong
,
Wang, ZhiRu
في
Collaborative filtering
,
Complexity
,
Computational Intelligence
2023
Representation learning of users and items is the core of recommendation, and benefited from the development of graph neural network (GNN), graph collaborative filtering (GCF) for capturing higher order connectivity has been successful in the recommendation domain. Nevertheless, the matrix sparsity problem in collaborative filtering and the tendency of higher order embeddings to smooth in GNN limit further performance improvements. Contrastive learning (CL) was introduced into GCF and alleviated these problems to some extent. However, existing methods usually require graph perturbation to construct augmented views or design complex CL tasks, which limits the further development of CL-based methods in the recommendation. We propose a simple CL framework that does not require graph augmentation, but is based on dropout techniques to generate contrastive views to address the aforementioned problem. Specifically, we first added dropout operation to the GNN computation, and then fed the same batch of samples twice into the network for computation. Using the randomness of dropout, a pair of views with random noise was obtained, and maximizing the similarity of the view pairs is set as an auxiliary task to complement the recommendation. In addition, we made a simple modification to the computation of the GNN to alleviate the information loss due to embedding smoothing by means of cross-layer connected graph convolution computation. We named our proposed method as Simple Contrastive Learning Graph Neural Network based on dropout (SimDCL). Extensive experiments on five public datasets demonstrate the effectiveness of the proposed SimDCL, especially on the Amazon Books and Ta-Feng datasets, where our approach achieves 44% and 43% performance gains compared to baseline.
Journal Article
Ripple-associated high-firing interneurons in the hippocampal CA1 region
By simultaneously recording the activity of individual neurons and field potentials in freely behaving mice, we found two types of interneurons firing at high frequency in the hippocampal CA1 region, which had high correlations with characteristic sharp wave-associated ripple oscillations (100-250 Hz) during slow-wave sleep. The firing of these two types of interneurons highly synchronized with ripple oscillations during slow-wave sleep, with strongly increased firing rates corresponding to individual ripple episodes. Interneuron type I had at most one spike in each sub-ripple cycle of ripple episodes and the peak firing rate was 310±33.17 Hz. Interneuron type II had one or two spikes in each sub-ripple cycle and the peak firing rate was 410±47.61 Hz. During active exploration, their firing was phase locked to theta oscillations with the highest probability at the trough of theta wave. Both two types of interneurons increased transiently their firing rates responding to the startling shake stimuli. The results showed that these two types of high-frequency interneurons in the hippocampal CA1 region were involved in the modulation of the hippocampal neural network during different states.
Journal Article